How does the LinkedIn algorithm define what content to display to you? At what moment? Behind the “black box”, there is a fairly basic logic. I’ll explain it to you.
How does the LinkedIn News Feed work?
LinkedIn is a social network. And like the majority of social networks today, their business is based on advertising.
The business model of advertising is to sell the attention of users to brands looking to promote their services, products or content.
We can therefore imagine that if they sell attention, the more they have, the greater their potential gains. This is the whole model of social networks like LinkedIn: getting more of your attention to earn money 🤑.
A social network must therefore make you spend the most time on its site or application.
To do this, they set up different mechanisms:
Triggers
These are web mechanisms, especially present on social networks 🕸️, which are defined as “action triggers”. That is to say that the user will be prompted to perform an action on LinkedIn: read its notifications, respond to a message …
External triggers
They announce to the user the action to be taken by placing the information in his field of vision 👀. Often associated with calls to action, the messages are directive, the words are used in this way: do this + now.
Example: a button “download the application now” or “watch the demo”.
We find these types of triggers on LinkedIn Ads.
The purpose of external triggers is to lead the user somewhere, to a particular page for example.
Internal triggers
Internal triggers are habit triggers, meaning that the user is already active on LinkedIn and scrolls through the news feed or visits his notifications. They do this because they have seen internal triggers, pop-ups indicating that so-and-so has replied to their post, or not habit.
The purpose of the internal trigger is to keep the user on the professional social network.
Notifications and emails to get you back on the network
Email notifications are clearly an incredible technique. When you sign up for LinkedIn, the network asks you to accept a small number of settings, all checked in advance, one of which is that you agree to receive your email notifications.
Everyone checks their email several times a day. Even me, even though I never receive emails, I check my mailbox. 😂
So, if you’re not on LinkedIn, the network will write you, several times a day, a little automated email that tells you that you missed some key information about your day, that Virginie commented on your post or that Jules changed jobs… This will arouse your curiosity and make you want to click.
Cognitive biases
Cognitive biases are psychological deviations. They lead to a distorted perception of reality. This form of thinking influences our actions, because it transforms our thoughts, judgments and appreciations.
Here are two examples of cognitive biases to help you understand:
- Fad = The number of people performing a behavior encourages you to do it.
Everybody went to see the last Spider Man at the cinema and loved it, so you will see it even if you are not interested in it, and you will even end up loving it without really knowing why, it’s the group effect or the fashion effect. Marketing strategies are very much inspired by this to make a product very popular, very quickly.
- Belief bias = We define something as true, because it aligns with our beliefs, without really knowing why.
80% of LinkedIn users believe that the network contains relevant and true information. That is, they trust LinkedIn, because in their established beliefs, it is a professional social network, and professional = relevant.
The biases that LinkedIn uses are very well known, such as the “Fear Of Missing Out” or “FOMO” that encourage us to come back and see what we would have missed.
The Random Reward
This is my favorite brain hack. 🧠
At least, it’s the one I find most interesting.
You never quite know what you’ll find when you scroll through a LinkedIn news feed. Every once in a while a particularly relevant post creates a dopamine (the happiness hormone) rush in us. Like any good primate, we look for that dopamine level in other content by scrolling even more.
It is the random reward that leads us to scroll. That is, we never know if we will find an interesting LinkedIn post while scrolling, and it is the fact of not knowing, hence the term “random”, that makes us addicted to the never-ending news feed.
I don’t go into detail about these psychological mechanisms. I invite you to read “Hooked” by Nir Eyal, a best-selling reference on the subject.
What we are particularly interested in is the following 👇 and how the LinkedIn algorithm, shows us relevant posts.
How do you beat LinkedIn algorithm : Emotions and engagement rate on a post
A very large majority of the audience on social networks is silent. This is one of the major pivots of Twitter: they initially thought that everyone was going to Twitter. When they looked at their data, they realized that the majority of people were looking to follow content without creating or interacting with it.
Still, it’s worth noting that the number of people who post on the networks is still growing, there is and will always be a majority of “content consumers” and a minority of “content creators”.
By observing viral posts on social networks we notice two things:
- They often have a strong emotional impact (this is why videos of kittens are always so popular on the web).
- They have a high engagement rate (ratio of comments + likes / views).
- They are well done, either well written or well illustrated.
These three observations are directly linked: content with a strong emotional impact has a greater chance of making us react (whether this impact is negative or positive in the process).
However, as we said before, LinkedIn (and other social networks in general) aims to make you feel intense emotions, which will release hormones in your body.
But the LinkedIn algorithm being (still today) incapable of feeling these emotions and determining the quality of content, it will be based on the emotions felt by people before you.
And is it measured? Via the engagement rate of course!
How to engage on LinkedIn: Likes
To measure your performance, we’re going to base it on the engagement of your community. Okay, you’ve got that right. But did you know that each action brings a different score?
The person who is going to read you can choose the reaction he wants. He will have the choice of 6 icons (or emojis). But be aware that the algorithm will give you +2% of reach and 3% to the one who will click on one of the reactions (yes it is also beneficial for you to go and like other creators’ content on LinkedIn). You can also find content that interests you by typing your keywords directly in the search bar).
Share a post
This is an interaction that is used a little less than the others. However, if you are going to share a post that you liked, there are some rules to follow. Indeed, you will have to add a bit of your personal brand. What do we mean by that? It’s pretty simple.
In order to personalize a bit a content you want to share, feel free to :
- Add value. Write at least 150 words so that the algorithm understands that you took time to add content.
- Mention the name of the person who wrote the post. This will also allow them to reply to you and improve your exchanges.
- Don’t hesitate to add hashtags.
- Respond systematically within the first hour if you receive comments.
Understanding LinkedIn algorithm : Comments
Undeniably, comments will get you the most points on your post. LinkedIn loves interaction. That’s what you have to look for. When you have a comment under your content, you multiply its reach by 4 compared to a like and by 7 if it was published in the first two hours 🤯.
There’s a little rule to this though: the comment generated under your post must be more than 5 words.
Tag on LinkedIn
We don’t recommend tagging someone in a post. You can do it, but you should (in principle) ask the person mentioned if he/she agrees to have his/her name mentioned.
On the other hand, don’t hesitate to answer him/her in the comments by mentioning his/her name if he/she asked you a question for example.
Are external links important on LinkedIn?
When you write your very long post, you may be tempted to insert external links (to your landing page for example).
We strongly advise against doing this, it is not a great way to please LinkedIn algorithm. Indeed, you are pushing a person to leave the platform, therefore, it will not please the algorithm of the professional social network much.
We’re not telling you not to do it, but to “cheat” 💔 a little LinkedIn to get your way. For example, you can either:
- Write your publication, put it online, modify it by adding your link.
- Or put your link in a comment so that network members can check it out.
Get your first customers this week
Take advantage of the power of Waalaxy to generate leads every day. Start prospecting for free, today.
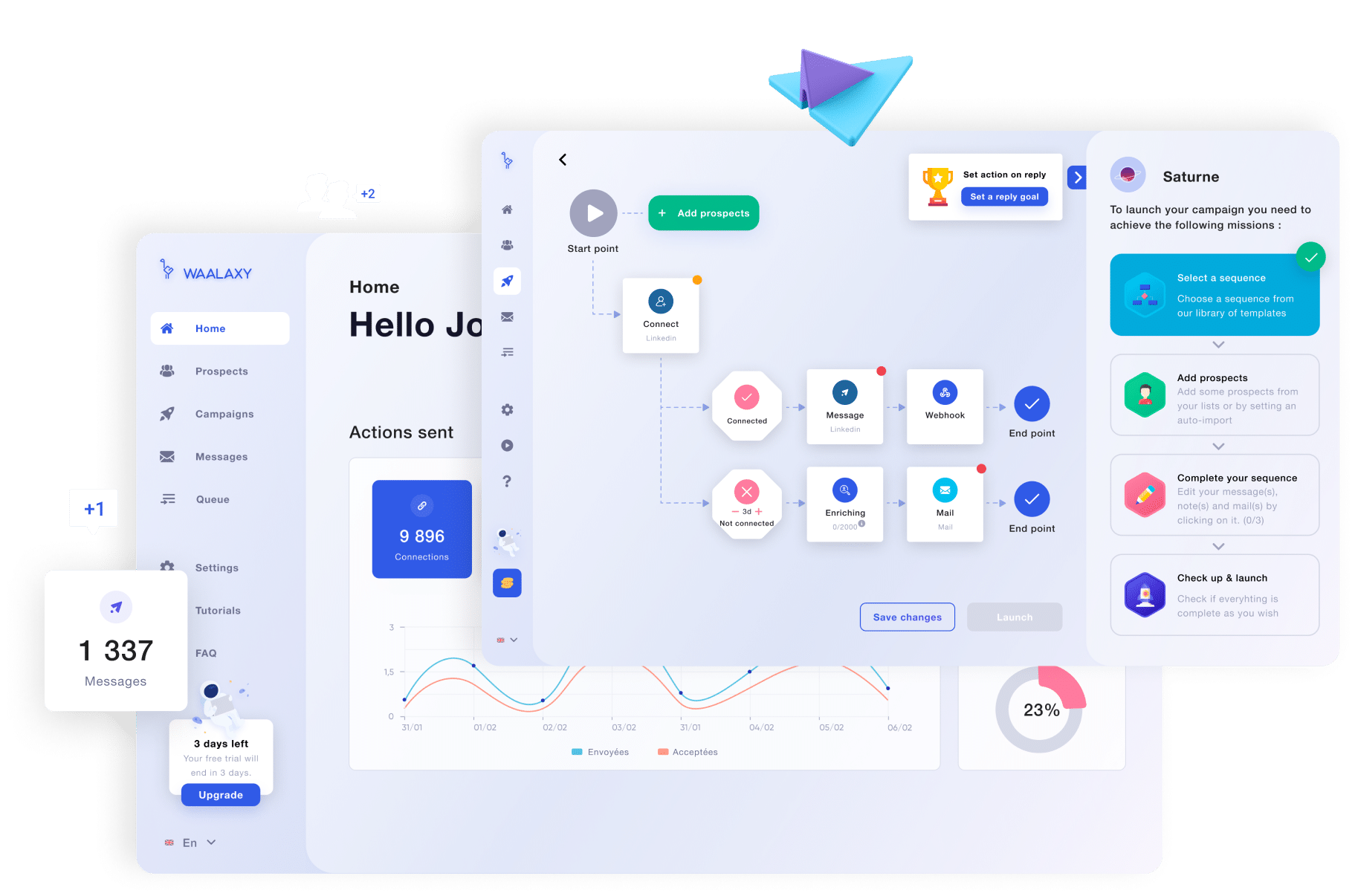
How does the LinkedIn algorithm work?
The higher the proportion of comments and likes / views, the more the content will be shown to a large audience.
The process works in the form of a spiral:
- When you publish your content, it’s displayed to a small part of your network, which acts as a sample. The sample size varies depending on the optimization of your post
- The algorithm observes what is the engagement rate on this sample, ie the rate of people who like and comment. This initial rate is essential because it largely influences the final reach of the post. Or rather the reverse: the algorithm considers that if there is no strong initial commitment, the content is not relevant. It is said to be measured between the first hour and the first three hours of content life.
- Based on this initial engagement rate, the algorithm widens the audience by prioritizing the people in your network, but especially the people in the network of those who engaged on your post, considered as “similar profiles”, who could therefore also be interested in your content. (Yes we tend to like the same things as people around us, culture, network, so to feel similar emotions on similar content).
- If the engagement rate continues to be similar, the post continues to grow in the number of views. This is what allows content with a high engagement rate to go viral.
There is an important criterion to take into account:
- As you can imagine, posting a comment is an act that requires much more involvement than a like. They do not have the same “emotional weight” at all, and therefore not the same weight for the algorithm. I would say that the ratio is between 10 and 20 (1 comment = 10 to 20 likes).
Other factors that go into the LinkedIn algorithm
We can say that the engagement rate far exceeds all the other criteria for distributing a publication.
However, certain other criteria will be able to influence (especially downward), the scope of a post:
- The number of hashtags used. The hashtag is like a “category” of the post for LinkedIn. If there is none, the algorithm cannot categorize it. If there are too many, it will consider that it is excessive and that you try to make it appear in all the categories.
- Emojis. You can use them to energize your publication and attract your reader.
- Network size: although it seems that this factor influences very little, it would seem that the size of your network can play on the initial sample of the post. (A study is planned to invalidate or confirm this hypothesis).
- Readability: a view on LinkedIn is just a person who passes on your post, without necessarily stopping. If your post is not readable, the likelihood of engagement is very low.
- Views on your latest publications. If your last posts have had a lot of views, LinkedIn will tend to enlarge the initial sample, considering that the quality of the post is more likely to be good.
- Outbound links. As we said, LinkedIn wants to keep users on the network to monetize them. Including links out of LinkedIn in your posts increases the chances of the user leaving LinkedIn. Publications containing an outgoing link are therefore devalued.
- Dwell time. You don’t think it means anything to you? Well, in fact, it’s simply the time a person spends on a post or on an article. And you understood it, the more this one, your reader, stays to read it, the more interesting it seems, which will give an indication to the algorithm of the platform.
Yes, that’s quite a lot of things that will have an impact on the LinkedIn algorithm.
What about the Social Selling Index (SSI) on LinkedIn?
In addition to Dwell Time, we have another new word in English? Well, yes, here it is more exactly your “sales score” on LinkedIn. The SSI is what will allow you to measure your own performance on the platform that is LinkedIn.
If you want to know it, you can go to this link.
So how is your SSI calculated? LinkedIn uses 4 criteria that it will score out of 25 each (if you have done your calculations correctly, you have a score out of 100):
- Professional presence or brand.
- How you’re doing connecting with the right people.
- Putting together relevant content.
- Developing your network.
This will allow you to measure your sales performance and identify some areas for improvement. So it’s a very powerful tool that will allow you to track your performance and help you generate 💰 sales opportunities.
Conclusion of the article What is the LinkedIn Algorithm
Because the LinkedIn algorithm is a black box, these explanations are based on observations made by the LinkedIn community over time. The algorithm is likely to evolve and the influence of certain criteria remains unknown. 🚀
How LinkedIn’s algorithm works
As said before, we are constantly trying to understand the secrets of LinkedIn’s algorithm (and of all other social networks for that matter). What we do know is that it will decide which content to put forward rather than another.
It’s the one who will decide what best fits a user, what is the strong added value that it can bring him or her, or even the one who will decide the relevance of a publication.
If you manage to hack it in one way or another, then your content will be put forward according to the targets you have set up.
Faq of the article on the LinkedIn algorithm
LinkedIn Algo : Relationship suggestions
Beyond the visibility of a publication, when LinkedIn proposes to add a new relationship in your network, it is also its algorithm that manages this. Of course, it is based on your previous searches.
Let’s say you’ve visited several male profiles specializing in the food industry. This will make it more likely that LinkedIn’s algorithm will suggest these types of profiles for you to add in the future. Indeed, it offers you results based on your previous searches (so it tries to answer what you ask).
How to make a successful post on LinkedIn?
When we talk about creating content on the network, we are talking about a few rules that must be met to ensure that you offer concrete content and reach your targets.
First, we need to identify:
- Who are you targeting ? (your targeting should be B2b).
- What is the message you want to convey?
Then, what is your marketing strategy?
- Is your goal to increase the visibility of your brand?
- Is your goal to generate traffic to your website?
- Maybe your goal is to sell your solutions?
Next, if you have already defined the type of content you want to share, ask yourself:
- Do you offer useful and/or value-added content?
- Is this content good for your branding and brand awareness?
LinkedIn algorithm tips: When should you publish on LinkedIn?
Wondering when to publish with your LinkedIn page? Everyone asks this question. As if there is a secret recipe or a magic potion to develop your visibility faster. Honestly, it will depend on your target, and also on the tenacity of your competition.
You have to stand out, by not publishing at the same time as everyone else (Tuesday and Thursday afternoon have been identified as the best days, but then everyone else publishes, so you don’t get more visibility).
So, my advice is to do A/B tests, to check the reactivity of your audience on different time slots, and then see for yourself.
Still, avoid the weekends. 🧠
How do I get comments on my publications?
As you know, when you are on a social network, the most important thing is the engagement. That is, the action that the user will do with a post. There are the :
- Likes,
- Shares,
- Comments.
Yet these three elements are not equal. We’ll explain right away. Let’s say you’ve written a post and you want it to be shown to as many people as possible (which is what everyone would want). Some people in your audience might give a reaction. They will allow you to gain a certain score on it.
On the other hand, a comment is the Holy Grail 🏆. If you receive comments, the algorithm will make sure to favor you and show your publication to as many people as possible.
Do you want to receive feedback? No problem, just ask for it without actually asking for it. What? You can ask a question, ask your readers for feedback. And… Of course they’ll answer you. Pretty cool, right?
How often should I post on LinkedIn?
Here, the answer becomes clearer. If your goal is to achieve a real marketing and editorial strategy on the long term, then we advise you to publish 3 times a week.
This way, you will create a name for yourself, continue to expand your audience and find prospects, while ensuring the loyalty of the acquired audience.
Creator mode on LinkedIn
Didn’t think of that? And yet, it could be very useful to you. To explain it in simple terms, thanks to the LinkedIn creator mode, you can become an “influencer”. Your “connect” button turns into a “follow” button, your relationships are changed by subscribers (yes like if you were on Instagram).
The biggest advantage of enabling this mode? You will be able to discover content by some creators and be able to interact with them. Remember, when you comment on posts, you also gain reach.
Which communication strategy works best on LinkedIn?
But then, to write 3 times a week, find relevant topics and continue to be inspired, how do you do it?
It sounds complicated, but it can be very simple,
There is a technique called TOFU-MOFU-BOFU,
That is, you define a primary target, a secondary target and a broad target and you will oscillate between these 3 audiences.
To put this strategy in place, follow this link > Creating a LinkedIn publishing strategy.
Understanding the LinkedIn algorithm is all about taming it 😉